The growing variability in hydrological regimes and the more frequent and intense extreme events necessitate a transition from conventional rule-based reservoir management to adaptive operations. This transition can be facilitated by harnessing improved hydro-meteorological forecasts and implementing feedback and feedforward control strategies.
While the importance of assessing hydrological forecast value for reservoir operation has long been recognized and studied, new opportunities and challenges are now emerging thanks to the availability of a wealth of hydro-meteorological forecast products over different time scales. These products often include information on uncertainty via ensemble forecasts, which is the current standard in operational forecasting.
When multiple forecasts from different systems are available, users should address a number of challenges, including the selection of the forecast product, the lead time, the variable aggregation, the bias correction, and how to cope with the forecast uncertainty. Usually, these choices are reservoir-specific and based on the operators’ experience, often lacking a transparent reporting of operational rules and guidelines to support such critical choices.
Within CLINT, we explore the potential for advancing forecast-informed reservoir operations via Reinforcement Learning (RL) algorithms. Our RL approach is evaluated using the Lake Como system in Northern Italy, a regulated lake primarily operated for flood control and water supply. The sub-alpine basin of the lake is characterized by mixed slow and fast dynamics resulting from the snow- and rain-dominated hydrology. In this context, forecasts over short and seasonal time scales may be valuable, and today the lake operator has access to different forecast products: short-term (i.e., 60 hours lead time) deterministic forecasts produced with locally calibrated models as well as the sub-seasonal and seasonal forecasts of the Copernicus Emergency Management Service’s European Flood Awareness System.
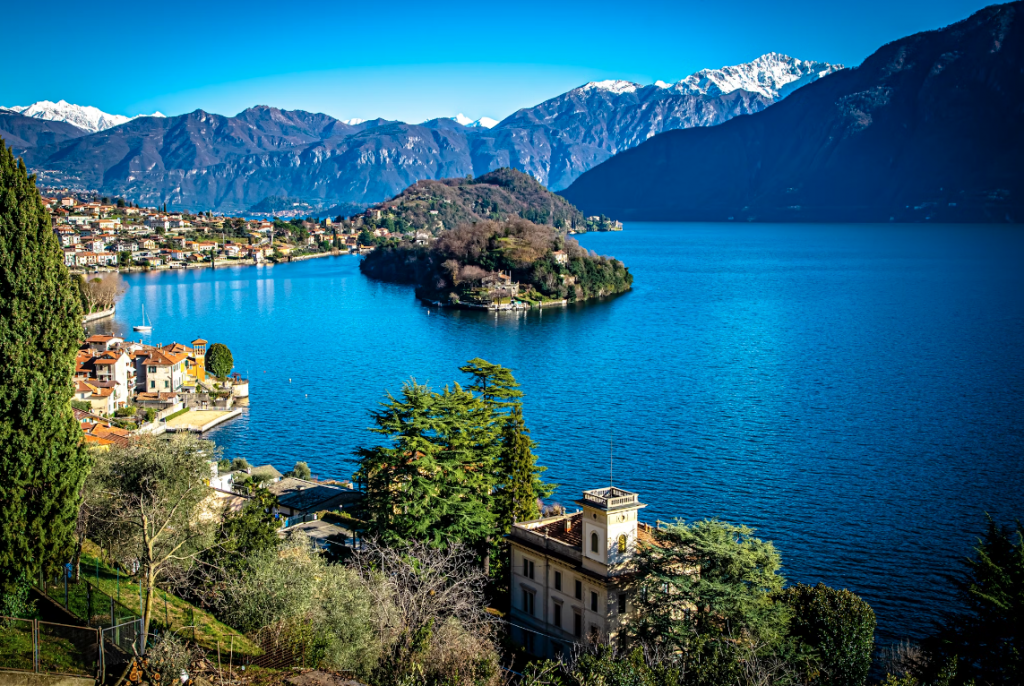
On Monday, May 20, 2024 we were at the 2024 World Environmental & Water Resources Congress presenting our results within the lecture ‘Mastering Multi-scale Ensemble Forecasts Using Reinforcement Learning for Advancing Forecast Informed Reservoir Operation‘, Speaker Matteo Giuliani.
Contributors: Andrea Ficchì, Andrea Castelletti, Davide Spinelli and Dennis Zanutto from Politecnico di Milano.
This study explores the potential of Reinforcement Learning (RL) algorithms to advance forecast-informed reservoir operations. The approach is evaluated using the Lake Como system in Northern Italy, which is primarily operated for flood control and water supply. The sub-alpine basin exhibits mixed slow and fast dynamics due to snow- and rain-dominated hydrology, making short-term and seasonal forecasts valuable.