Speaker: Matteo Giuliani, assistant professor, Politecnico di Milano
Moderator: Andrea Toreti, senior scientist, European Commission, Joint Research Centre
To join the webinar, register here
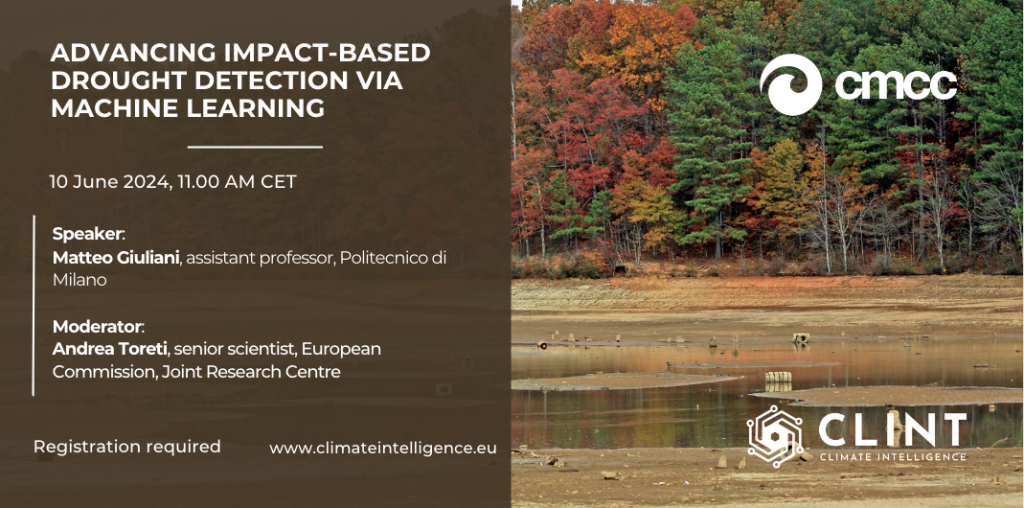
Abstract
Drought is a slowly developing natural phenomenon that can occur in all climatic zones and propagates through the entire hydrological cycle with long-term socio-economic and environmental impacts. Intensified by anthropogenic climate change, drought has become one of the most significant natural hazards in Europe. Different definitions of drought exist, i.e. meteorological, hydrological, and agricultural droughts, which vary according to the time horizon and the variables considered. Just as there is no single definition of drought, there is no single index that accounts for all types of droughts. Capturing the evolution of drought dynamics and associated impacts across different temporal and spatial scales still remains a critical challenge.
In this talk, we discuss the role of Machine Learning for advancing impact-based drought detection. Our main goal is the identification of relevant drivers of observed drought impacts (e.g., water deficits or crop stress) from a pool of candidate hydro-meteorological predictors. The selected predictors are then combined into an index representing a surrogate of the drought impacts in the considered area. To support this task, we developed a ML pipeline that integrates (1) a novel dimensionality reduction method that allows an interpretable aggregation of spatially distributed drivers, (2) feature extraction techniques including both filters and wrappers to select the most informative and non-redundant information, and (3) existing and new causal inference algorithms for verifying the causal links between the selected drivers and the target impacts.
Our new indexes advance state-of-the-art drought monitoring practices, which often rely on
standardized drought indexes that are poorly correlated with drought impacts, and provide reliable projections of drought impacts’ trends under different climate change scenarios. Several real-world examples will be used to provide a synthesis of recent applications of our methodology in case studies featuring diverse hydroclimatic conditions, variable levels of data availability, and increasing spatial domain from single river basins to a pan-European analysis.